| 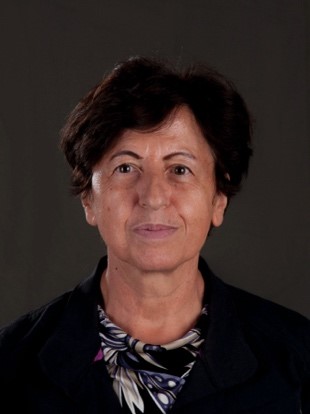 |
Applying Machine Learning to Securing Cellular Networks
Elisa Bertino
Purdue University, USA
|
Abstract: Cellular network security is more critical than ever, given the increased complexity of these networks and the numbers of applications that depend on them, including telehealth, remote education, ubiquitous robotics and autonomous vehicles, smart cities, and Industry 4.0. In order to devise more effective defenses, a recent trend is to leverage machine learning (ML) techniques, which have become applicable because of today advanced capabilities for collecting data as well high-performance computing systems for training of ML models. Recent large language models (LLMs) are also opening new interesting directions for security applications. In this talk, I will first present a comprehensive threat analysis in the context of 5G cellular networks to give a concrete example of the magnitude of the problem of cellular network security. Then, I will present two specific applications of ML techniques for the security of cellular networks. The first application focuses on the use of natural language processing techniques to the problem of detecting inconsistencies in the "natural language specifications" of cellular network protocols. The second application addresses the design of an anomaly detection system able to detect the presence of malicious base stations and determine the type of attack. Then I'll conclude with a discussion on research directions.
Biography: Elisa Bertino is a Distinguished Samuel Conte professor of Computer Science at Purdue University. She serves as Director of the Purdue Cyberspace Security Lab (Cyber2Slab). Prior to joining Purdue, she was a professor and department head at the Department of Computer Science and Communication of the University of Milan. She has been a visiting researcher at the IBM Research Laboratory in San Jose (now Almaden), at Rutgers University, at Telcordia Technologies. She has also held visiting professor positions at the Singapore National University and the Singapore Management University. Her recent research focuses on security and privacy of cellular networks and IoT systems, and on edge analytics for cybersecurity. Elisa Bertino is a Fellow member of IEEE, ACM, and AAAS. She received the 2002 IEEE Computer Society Technical Achievement Award for “For outstanding contributions to database systems and database security and advanced data management systems”, the 2005 IEEE Computer Society Tsutomu Kanai Award for “Pioneering and innovative research contributions to secure distributed systems”, the 2019-2020 ACM Athena Lecturer Award, and the 2021 IEEE 2021 Innovation in Societal Infrastructure Award. She received an Honorary Doctorate from Aalborg University in 2021 and an Honorary Research Doctorate in Computer Science from the University of Salerno in 2023. She is currently serving as ACM Vice-president.
| 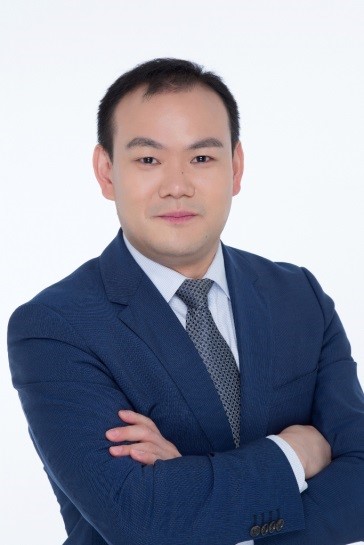 |
Composite DP-unbias: Bounded and Unbiased Composite Differential Privacy
Jinjun Chen
Swinburne University of Technology, Australia
|
Abstract: The most kind of traditional DP (Differential Privacy) mechanisms (e.g. Laplace, Gaussian, etc.) have unlimited output range. In real scenarios, most datasets have bounded output range. Users would then need to use post-processing or truncated mechanisms to forcibly bound output distribution. However, these mechanisms would incur bias problem which has been a long-known DP challenge, resulting in various unfairness issues in subsequent applications. A tremendous amount of research has been done on analyzing this bias problem and its consequences, but no solutions can solve it fully.
As the world first solution to solve this long-known DP bias problem, this talk will present a new innovative DP mechanism named Composite DP-unbias. It will first illustrate this long-known bias problem, and then detail the rational of the new mechanism and its example noise functions as well as their implementation algorithms. All source codes are publicly available on Github for any deployment or verification.
Biography: Dr Jinjun Chen is a Professor from Swinburne University of Technology, Australia. He holds a PhD in Information Technology from Swinburne University of Technology, Australia. His research interests include data privacy and security, cloud computing, scalable data processing, data systems and related various research topics. His research results have been published in more than 300 papers in international journals and conferences. He received various awards such as IEEE TCSC Award for Excellence in Scalable Computing and Australia’s Top Researchers. He has served as an Associate Editor for various journals such as ACM Computing Surveys, IEEE TC, TCC and TSUSC. He is a MAE (Academia Europea) and IEEE Fellow (IEEE Computer Society). He is Chair for IEEE TCSC (Technical Community for Scalable Computing).
| 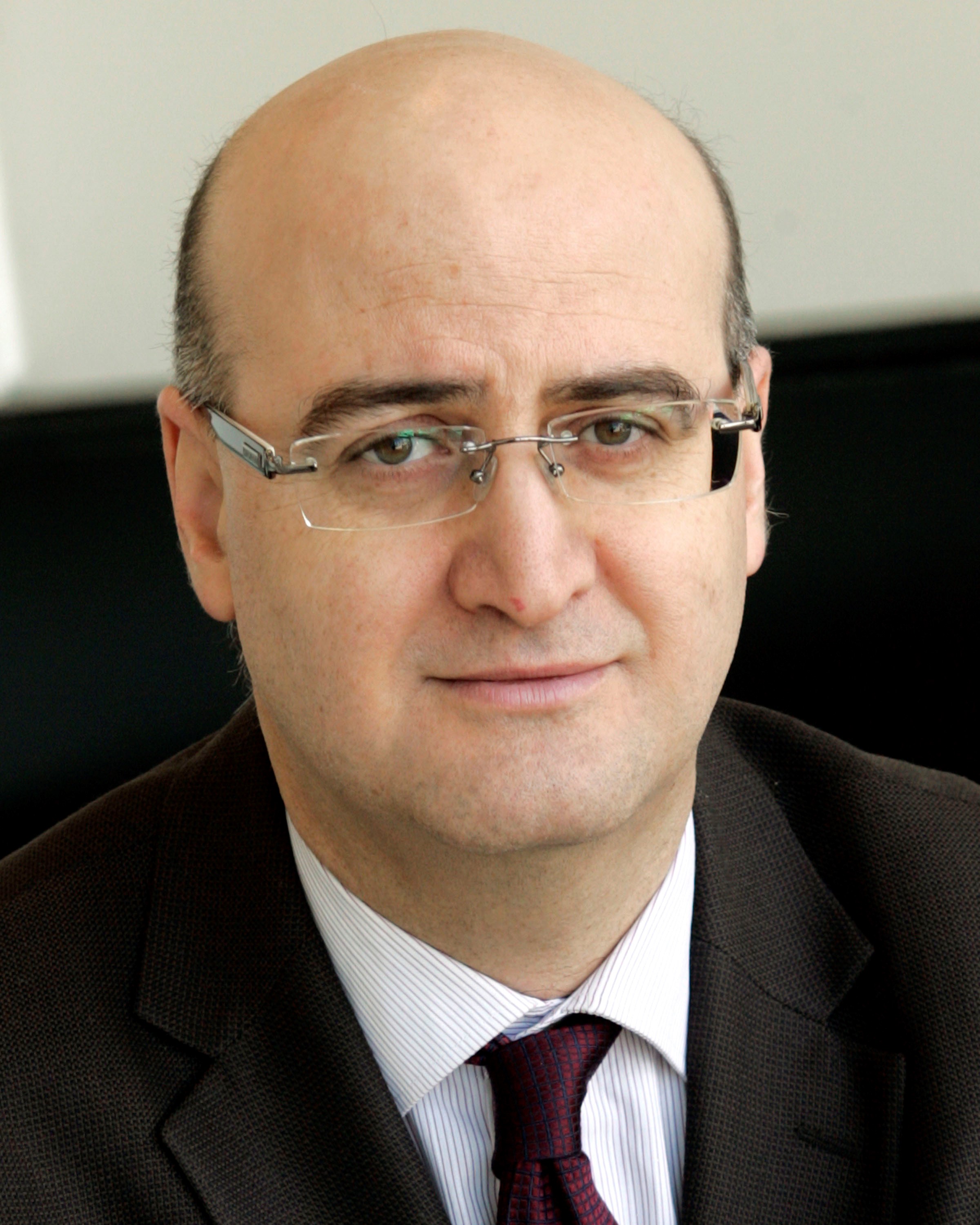 |
The Super Neuron Model -- New Generation Machine Learning and Applications
Moncef Gabbouj
Tampere, University, Finland
|
Abstract. Deep Learning is great as it has outperformed many traditional approaches in numerous fields. However, DL comes at a price of high computational cost and follows mostly a Blackbox approach. Striving towards Green Learning, we will propose and discuss Operational Neural Networks (ONNs) as more efficient alternatives to conventional Convolutional Neural Networks (CNNs). ONNs can perform any linear or non-linear transformation with a proper combination of “nodal” and “pool” operators. This is a great leap towards expanding the neuron’s learning capacity in CNNs, which thus far required the use of a single nodal operator for all synaptic connections for each neuron. This restriction has recently been lifted by introducing a superior neuron called the “generative neuron” where each nodal operator can be customized during the training to maximize learning. As a result, the network can self-organize the nodal operators of its neurons’ connections. Self-Organized ONNs (Self-ONNs) equipped with superior generative neurons can achieve diversity even with a compact configuration. A novel approach to enforce diversity in ANN will also be discussed. We shall explore several applications of neural network models equipped with the generative and the superior neuron.
Biography. MONCEF GABBOUJ received his BS degree in 1985 from Oklahoma State University, and his MS and PhD degrees from Purdue University, in 1986 and 1989, respectively, all in electrical engineering. Dr. Gabbouj is a Professor of Information Technology at the Department of Computing Sciences, Tampere University, Tampere, Finland. He was Academy of Finland Professor during 2011-2015. His research interests include Big Data analytics, artificial intelligence, machine learning, pattern recognition, and video processing and coding. Dr. Gabbouj is a Fellow of the IEEE and member of the Academia Europaea and the Finnish Academy of Science and Letters. He is the past Chairman of the IEEE CAS TC on DSP and committee member of the IEEE Fourier Award for Signal Processing. He served as associate editor and guest editor of many IEEE, and international journals and Distinguished Lecturer for the IEEE CASS. Dr. Gabbouj served as General Chair of IEEE ICIP 2024, ISCAS 2019, ICIP 2020, and ICME 2021. Gabbouj is Finland Site Director of the USA NSF IUCRC funded Center for Big Learning and led the Artificial Intelligence Research Task Force of Finland’s Ministry of Economic Affairs and Employment funded Research Alliance on Autonomous Systems (RAAS).
|